Demystifying artificial intelligence: Fitting AI into e-commerce
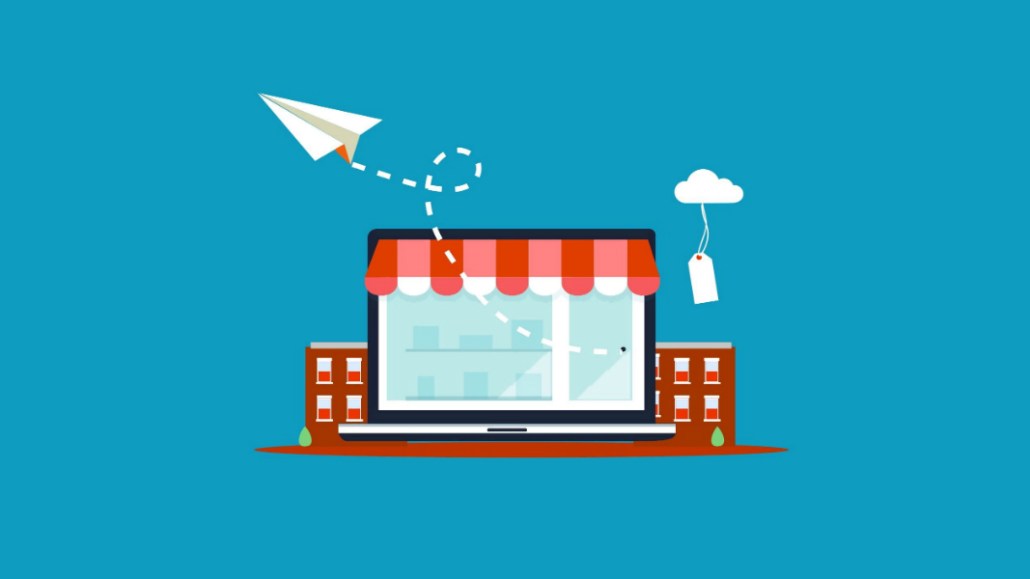
Rob May, founder and CTO, Dianthus
Artificial intelligence produces an increasing amount of hype. Yet, many in the e-commerce space don’t know exactly what it is or how it can be used to grow a customer base, make marketing decisions and achieve growth.
AI is primarily used to predict something, automate a task or classify data into predefined buckets. When used correctly, AI can be a powerful tool for scaling an e-commerce business. Several existing AI and machine learning tools can be easily installed on e-commerce websites to begin working right away.
However, with the rise in AI solutions, there are strong solutions, and then there are less than robust approaches. Some overpromise and under-deliver; in either case, finding credible AI tools that are effective and match the marketing team’s needs is essential to success in the digital commerce world. In pursuit of that right fit, there are several steps e-commerce leaders can take to strengthen their bets on strong AI outcomes.
Identifying how AI can help while being mindful of red flags
The first thing a business should ask is how AI can best help them. Reviewing solutions and deciding what’s most important will help companies determine what they want AI to do for them and allow them to research the best tool. However, identifying the good eggs from the bad is the next challenge.
It’s important to ask detailed questions about the accuracy of the models a given AI solution brings to bear and how much those models can actually contribute to outcomes.
A red flag when it comes to models appears if the system in play uses data that is inappropriately matched to the problem the marketing team is trying to solve. Successful AI approaches start with the business use case and what information a human needs to analyze the problem under consideration. A machine will most likely need the same data. However, AI companies often use the data to which they have access, whatever that might be, and not the best data for solving the problem. For marketing teams assessing a solution, it’s essential to consider if there are public data sets to leverage or if it’s possible to purchase relevant data sets to help solve the problem. If the tool only relies on the customer to provide the data, the business needs to ensure they have the correct data inputs to fix the problem.
To that end, if the software provider needs the business’ data to complete the analysis, which is usually the case, then what rights do they have to it if the partnership is canceled? A lack of data rights knowledge is a red flag.
Ultimately, it’s important to note that weak data resources can signify a less than ideal provider.
Selecting the right tools and interviewing prospective companies
Once the e-commerce business has identified its needs and what to be mindful of, it’s time to select a tool that fits those needs and set up a demo. When setting up the demo, it’s important to question the company and get more information on their technology and how they operate.
Some questions to ask include: How is the AI model trained? Is it trained on data the e-commerce business provides or on some other type of data? Good models are trained on appropriate datasets — they must be relevant to the problem being solved and large enough to have predictive value.
Another question is, does the data need to be labeled? If so, by whom? Machine learning models need data inputs that have labels explaining what they are. The more labeled data a company has to train on, the better the model will usually work. But labeling data is work, and someone has to do it. A business should understand if that falls on them and their team.
Furthermore, what does the post-integration work look like? Unfortunately, AI isn’t magic and sometimes requires ongoing attention. But, how much and is it worth the return? A company can’t just deploy it and ignore it. The team at work on the program must clearly understand the steps that will follow the moment they (and their partner) throw the AI switch.
Finally, the shortlist of questions to ask includes: How much data is required to begin a project? One primary reason AI projects fail is that there isn’t enough data to train an accurate model. Make sure to ask the right questions to uncover this before getting too far down the path.
After making a decision, marketers must remember that AI sometimes requires a cultural change. The way a team works before AI and with implemented AI may be different. AI products sometimes give probabilities rather than always providing binary answers. Training teams to understand the difference and adapt accordingly is crucial.
With so many great tools on the market, figuring out the right ones for a business is difficult. Artificial intelligence has strong potential to accelerate e-commerce businesses, given the right approach and implementation. By focusing on the right things and ignoring the overhyped tools that don’t provide any AI benefits, e-commerce businesses can be set up for growth now and in the future.
Sponsored By: Dianthus